Solar panels are at the forefront of the renewable energy revolution, offering sustainable solutions for residential, commercial, and industrial energy needs. As global demand grows, ensuring the efficiency and longevity of solar panels is paramount. A groundbreaking machine-learning method, recently developed by a researcher at Sweden’s Jönköping University, offers an innovative approach to identifying and diagnosing faulty solar panels using infrared thermography. This method not only promises enhanced operational efficiency but also highlights advancements in machine-learning applications in renewable energy systems.
Revolutionizing Solar Panel Monitoring with Machine Learning
The proposed method employs a hybrid local features-based approach designed to address limitations in traditional image processing methods. These conventional approaches are often sensitive to environmental factors such as haze, noise, scaling, and rotation. In contrast, this machine-learning model offers remarkable robustness and efficiency, achieving a training accuracy of 98% and testing accuracy of 96.8%.
Methodology Overview
The new method starts by capturing infrared thermographs of solar panels. Infrared thermography is an advanced diagnostic tool that visualizes heat patterns, making it ideal for identifying hotspots and other anomalies in PV systems. The methodology involves:
① Preprocessing Thermographs: Dehazing algorithms and contrast adjustments are applied to remove noise and improve image quality. Each thermograph is divided into smaller sub-thermographs (5×5 pixels) to facilitate localized analysis.
② Feature Extraction and Optimization: Local features are extracted using Gaussian and nonlinear methods, with only the most significant 80% retained for analysis. Unsupervised clustering algorithms, such as k-means, reduce the feature vector to 300 elements per thermograph, optimizing memory usage.
③ Classification: A support vector machine (SVM) classifier is trained on these optimized feature vectors using a 5-fold cross-validation technique. The model classifies solar panels into three health categories: healthy, hotspot, and faulty.
Key Findings and Performance Metrics
The method was tested on a 44.24 kW rooftop PV system in Lahore, Pakistan, featuring crystalline silicon (c-Si) modules. The system operated under ambient temperatures of 32°C to 40°C, wind speeds of 6.9 m/s, and irradiance levels of 700 W/m². Highlights from the study include:
· Precision, Recall, and F1 Scores: The model achieved precision values of 92%, 100%, and 100%; recall values of 100%, 100%, and 90%; and F1 scores of 0.958, 1.0, and 0.947 for faulty, healthy, and hotspot classes, respectively.
· Comparison with Other Methods: The method outperformed most existing techniques, including texture and HOG-based methods, while being slightly surpassed by RB scale-invariant feature transform with a score of 98.66%.
The Growing Importance of Solar Panel Efficiency
The global market for solar panels is rapidly expanding, driven by advances in photovoltaic technology and growing interest in renewable energy. However, maintaining panel efficiency is a persistent challenge. Dust, shading, and equipment degradation can lead to reduced output and increased operational costs. This highlights the importance of innovative monitoring systems, such as the machine-learning approach discussed here.
Comparing Solar Panel Types and Technologies
To understand the impact of this monitoring method on the market, let’s compare several popular solar panel types and their applications:
Type | Power Rating | Features | Applications |
N-Type Topcon and BC Solar Panels | 580-620W | High efficiency, ideal for large installations | Commercial and utility-scale systems |
Roof Solar Panel | 440W - 480W | Full black or black frame for rooftop; Dual-sided light absorption for enhanced efficiency | Energy storage systems |
Double Glass Bifacial Solar Panel | 580W - 670W | Increased durability, suitable for harsh environments | Utility-scale projects |
The monitoring method’s ability to detect faults early can optimize the performance of these panels, ensuring maximum energy output over their lifespan.
Price Analysis: 2024 Hot Selling Wholesale Solar Panels
Understanding pricing trends is crucial for businesses and consumers looking to invest in solar technology. For instance, the 580W 585w 590w 600w 605w 610w 620w 630w 635w 650w 660w 670w solar panel range caters to diverse energy needs with competitive pricing, making it a popular choice among installers. Similarly, 430W 435w 440w 445w 450w 460w 470w solar panel full black or black frame solar panels for home offer an aesthetic solution for residential applications.
Future Trends and Opportunities in Solar Panel Technology
Emerging trends in the solar industry, such as bifacial and double-glass panels, are reshaping how energy systems are designed. These innovations, coupled with advanced monitoring technologies, can significantly enhance the sustainability and efficiency of solar installations.
Integration with Energy Storage Systems
The rise of bifacial solar panels for energy storage systems highlights the synergy between efficient solar generation and reliable energy storage. These systems ensure uninterrupted power supply and optimize the use of renewable energy.
Technological Advancements in Manufacturing
Manufacturers are increasingly focusing on producing high-efficiency panels such as the 485w solar panel and 670w double glass solar panel, ensuring compatibility with the latest monitoring and diagnostic tools.
Conclusion
The innovative machine-learning method developed by Dr. Waqas Ahmed represents a significant leap forward in the monitoring and maintenance of solar panels. By addressing common challenges like noise and environmental interference, this approach ensures precise fault diagnosis and enhances system reliability.
As solar technology continues to evolve, integrating advanced monitoring systems will be essential to maximize efficiency and minimize costs. The future of renewable energy lies in combining cutting-edge technologies with sustainable practices, paving the way for a greener and more energy-efficient world.
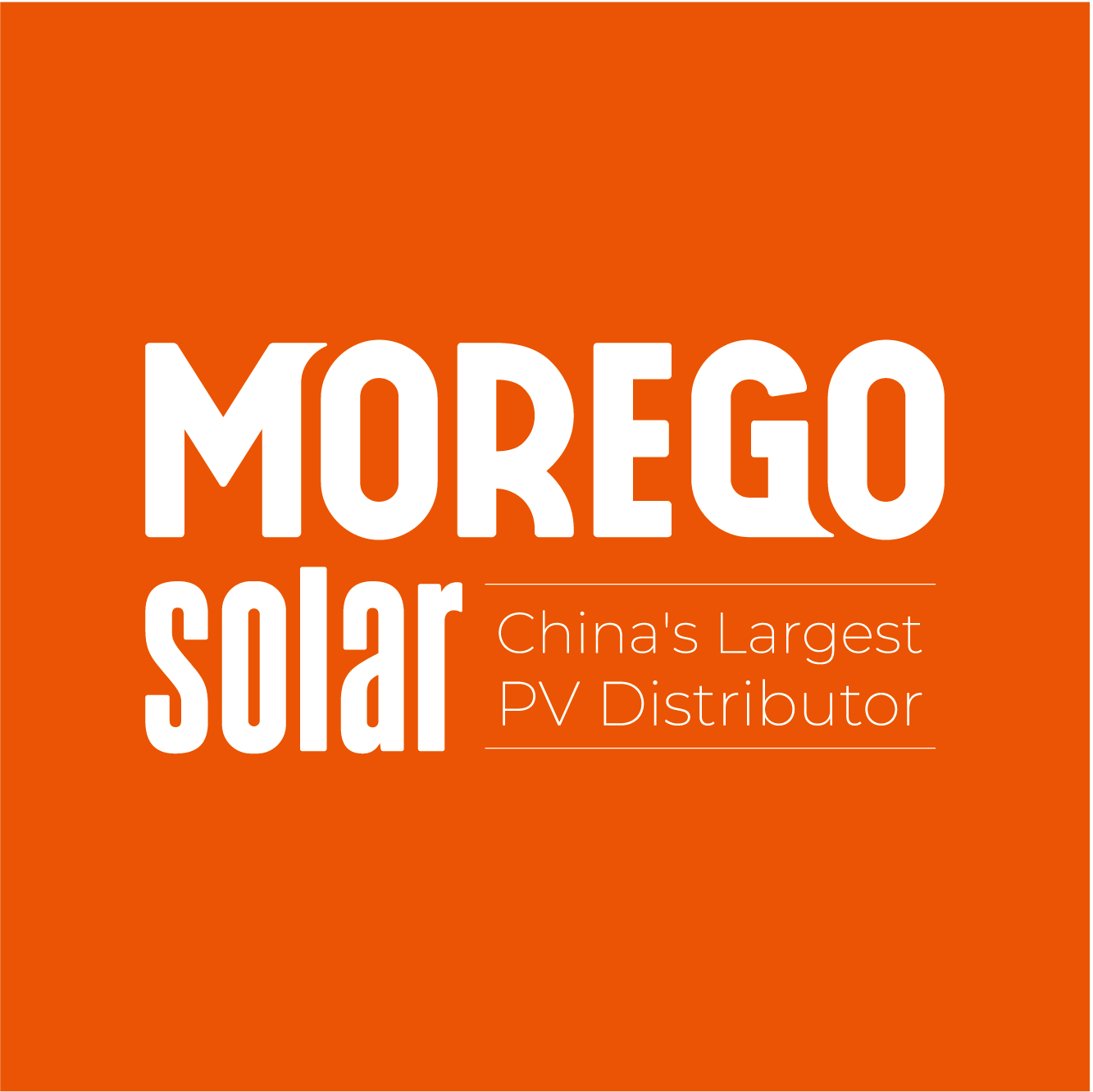